Object Detection
With over 200 new classes of objects, the Object Detection subsystem enhances Lightship's contextual awareness capabilities by creating semantically labeled 2D bounding boxes that dynamically update as real-world objects appear on-screen. For each bounding box, the subsystem processes the central square crop of the image, then makes an independent prediction for every subclass and returns the probability that the detected object belongs to each of them. Lightship Object Detection also provides the following model card which explains how detections were trained for person, a human hand, or a human face.
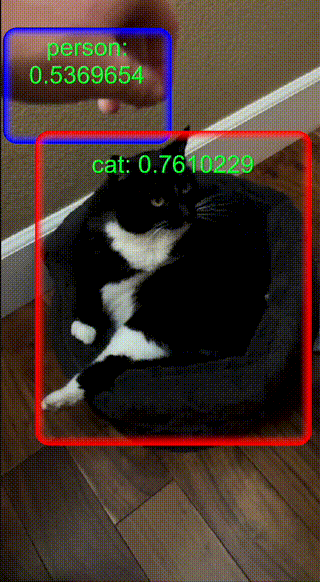
Basic Usage
By placing Lightship's ARObjectDetectionManager
in a scene and subscribing to the ObjectDetectionsUpdated
event, developers can receive realtime detection information in the form of XRDetectedObjects. You can also listen for the MetadataInitialized
event to receive the list of object classes when the model becomes available to use.
The frame rate of the ARObjectDetectionManager
can also be adjusted to save performance or detect objects at a faster rate.
Object Detection Categories
While the probability of each class is computed independently, the subclasses of each category are used to train the categorical classes they belong to. Because of this, objects can be detected as members of their categorical classes rather than their specific subclasses. For example, a French horn will return a high probability that the object is in the french horn
, brass instrument
, and musical instrument
classes.
Category | Subclasses |
---|---|
Aircraft | airplane, helicopter, hot air balloon, parachute, rocket |
Building features | door, door handle, window |
Car | car, taxi |
Outdoor furniture | barrel, bench, billboard, fire hydrant, flag, parking meter, sculpture, snowman, street light, traffic light, waste container |
Vehicle | vehicle, bicycle, boat, bus, car, cart, motorcycle, taxi, train, truck, wheel, wheelchair |
Water feature | fountain, swimming pool |
Accessories | backpack, glasses, handbag, umbrella |
Clothing | coat, dress, shirt, shorts, skirt, sock, suit, tie, trousers |
Footwear | footwear, roller skates |
Headwear | headwear, fedora |
Musical instrument | accordion, brass instrument, drum, flute, guitar, piano, string instrument, violin |
Brass instrument | french horn, saxophone, trombone, trumpet |
String instrument | banjo, cello, harp, guitar, violin |
Food | food, apple, banana, berry, bread, broccoli, cake, carrot, cheese, citrus, coconut, dessert, donut, egg, fast food, grape, hamburger, hot dog, ice cream, mushroom, pear, pizza, pumpkin, sandwich, sushi, tomato |
Berry | berry, raspberry, strawberry |
Citrus | citrus, grapefruit, lemon, lime, orange |
Dessert | dessert, cake, donut, ice cream |
Fast food | fast food, french fries, hot dog, pizza, hamburger, sandwich |
Pumpkin | pumpkin, squash |
Drink | drink, hot drink, juice |
Hot Drink | tea, coffee (recognized when in a cup) |
Appliances | hair dryer, microwave, oven, refrigerator, toaster |
Cooking pan | frying pan, pressure cooker, slow cooker, waffle iron, wok |
Indoor furniture | furniture, bed, chair, christmas tree, couch, curtains, poster, shelves, storage cabinet, table |
Jug | jug, teapot |
Lamp | lamp, candle |
Home features | bathtub, fireplace, sink, tap, toilet |
Miscellaneous items | book, bottle, bowl, box, cannon, chopsticks, coin, cup, flowerpot, fork, knife, pen, pillow, plate, potted plant, scissors, skull, spoon, tin can, toothbrush, wine glass |
Screen | screen, computer display, tablet, TV |
Sports ball | sports ball, football, rugby ball, tennis ball |
Sports equipment | baseball bat, baseball glove, frisbee, kite, paddle, skateboard, skis, snowboard, tennis racket |
Tech | camera, clock, computer keyboard, computer mouse, headphones, microphone, phone, remote, watch |
Toy | toy, doll, teddy bear |
Animal | animal, alpaca, bear, big cat, bird, camel, cat, cow, crocodile, deer, dog, dolphin, elephant, fish, frog, giraffe, goldfish, hippopotamus, horse, jellyfish, kangaroo, panda, parrot, pig, polar bear, rabbit, reptile, rhinoceros, seal, sheep, shellfish, squirrel, turtle, water bird, whale, zebra |
Big cat | cheetah, jaguar, leopard, lion, lynx, tiger |
Bird | bird, parrot, water bird |
Camelids | alpaca, camel, llama |
Crocodile | crocodile, alligator |
Deer | antelope, deer, moose |
Flower | flower, rose, sunflower |
Horse | donkey, horse, mule |
Insect | insect, butterfly |
Fish | fish, goldfish, jellyfish, manta ray, seahorse, shellfish, squid |
Reptile | reptile, crocodile, turtle |
Seal | seal, sea lion, walrus |
Sheep | goat, sheep |
Shellfish | crab, lobster, oyster, shrimp, snail, starfish |
Turtle | turtle, sea turtle, tortoise |
Water bird | duck, goose, swan |
Person | person, human face, human hand, |
Person Detection Model Card v0.4
Model Details
- Model last updated: 2024-02-29
- Model version: v0.4
- License: refer to the terms of service for Lightship.
Technical specifications
The object detection model returns a set of bounding boxes and reports the probability that the box is a person, a human hand, or a human face.
Intended use
Intended use cases
- Identifying people (more specifically, human hands or human faces) in an image.
- Querying the presence or absence of people, human hands, or human faces in an image.
Permitted users
Augmented reality developers through Niantic Lightship.
Out-of-scope use cases
This model does not provide the capability to:
- Track individuals
- Identify or recognise individuals
Factors
The following factors apply to all object detection provided in the Lightship ARDK, including person detection:
- Scale: objects / classes may not be detected if they are very far away from the camera.
- Lighting: extreme light conditions may affect the overall performance.
- Viewpoint: extreme camera views that have not been seen during training may lead to a miss in detection or a class confusion.
- Occlusion: objects may not be detected if they are covered by other objects.
- Motion blur: fast camera or object motion may degrade the performance of the model.
- Flicker: there may be a ‘jittering’ effect between predictions of temporally adjacent frames.
For person detection specifically, based on known problems with computer vision technology, we identify potential relevant factors that include subgroups for:
- Geographical region
- Skin tone
- Gender
- Body posture: certain body configurations may be harder to predict due to appearing less often in the training corpus.
- Other: age, fashion style, accessories, body alterations, etc.
Fairness evaluation
At Niantic, we strive for our technology to be inclusive and fair by following strict equality and fairness practices when building, evaluating, and deploying our models. We define person detection fairness as follows: a model makes fair predictions if it performs equally on images that depict a variety of the identified subgroups. The evaluation results focus on measuring the performance of the union of the human channels (person, human hand, and human face) on the first three main subgroups (geographical region, skin tone, and gender).
Instrumentation and dataset details
Our benchmark dataset comprises 5650 images captured around the world using the back camera of a smartphone, with these specifications:
- Only one person per image is depicted.
- Both indoors and outdoors environments.
- Captured with a variety of devices.
- No occlusions.
Images are labeled with the following attributes:
- Geographical region: based on the UN geoscheme with the merge of European subregions and Micronesia, Polynesia, and Melanesia:
- Northern Africa
- Eastern Africa
- Middle Africa
- Southern Africa
- Western Africa
- Caribbean
- Central America
- South America
- Northern America
- Central Asia
- Eastern Asia
- South Eastern Asia
- Southern Asia
- Western Asia
- Europe
- Australia and New Zealand
- Melanesia, Micronesia, and Polynesia
- Skin tone: following the Fitzpatrick scale, images are annotated from subgroup 1 to 6. Skin tone is a self-reported value provided by the person in each image.
- Gender: images are annotated with self-reported gender.
Metrics
The standard metric for evaluating object detection models -- and the one we use -- is Intersection over Union (IoU). It is computed as follows:
IoU = (overlap between predicted and g.t. boxes) / (union between predicted and g.t. boxes)
Reported IoUs are averages (mean IoU or mIoU) over images belonging to the referenced subgroup unless stated otherwise.
Fairness criteria
A model is considered to be making unfair predictions if it yields a performance (mIoU) for a particular subgroup that is three standard deviation units or more from the mean across all subgroups.
Results
Geographical evaluation
Average performance across all 17 regions is 78.74% with a standard deviation of 1.22%. All regions exhibit a performance in the range of [76.92%, 82.17%]. The maximum difference between the mean and the worst performing region is 1.83%, within our fairness criterion threshold of 3 standard deviations (3x1.22% = 3.65%).
Regions | mIoU | stdev | Number of images |
---|---|---|---|
Northern Africa | 78.26% | 15.04% | 301 |
Eastern Africa | 77.41% | 17.11% | 336 |
Middle Africa | 77.30% | 15.72% | 322 |
Southern Africa | 79.09% | 14.93% | 368 |
Western Africa | 79.04% | 13.26% | 364 |
Caribbean | 79.01% | 12.20% | 412 |
Central America | 79.44% | 13.79% | 415 |
South America | 78.39% | 14.21% | 397 |
Northern America | 79.09% | 13.00% | 335 |
Central Asia | 79.52% | 12.56% | 229 |
Eastern Asia | 77.60% | 15.37% | 346 |
South Eastern Asia | 77.86% | 14.86% | 333 |
Southern Asia | 79.34% | 12.15% | 353 |
Western Asia | 78.80% | 14.91% | 370 |
Europe | 79.40% | 13.14% | 320 |
Australia and New Zealand | 76.92% | 18.13% | 374 |
Melanesia, Micronesia and Polynesia | 82.17% | 11.08% | 75 |
Average (across all images) | 78.55% | 14.55% | 5650 |
Average (across regions) | 78.74% | 1.22% | - |
Skin tone evaluation results
Average performance across all six skin tones is 78.58% with a standard deviation of 0.24%. All skin tone subgroups yield a performance in the range of [78.23%, 78.97%]. The maximum difference between the mean and the worst performing skin tone subgroup is 0.34%, within our fairness criterion threshold of 3 stdevs (3x0.24% = 0.71%).
Skin tone (Fitzpatrick scale) | mIoU | stdev | Number of images |
---|---|---|---|
1 | 78.59% | 12.00% | 247 |
2 | 78.49% | 14.59% | 1919 |
3 | 78.61% | 14.39% | 1463 |
4 | 78.23% | 16.52% | 457 |
5 | 78.97% | 13.60% | 706 |
6 | 78.56% | 14.67% | 858 |
Average (across all images) | 78.55% | 14.55% | 5650 |
Average (across skin tones) | 78.58% | 0.24% | - |
Gender evaluation results
Average performance of all evaluated gender subgroups is 78.53% with a range [78.01%, 79.05%]. The difference between the average and the worst performing gender is 0.52%, within our fairness criterion threshold of 3 stdevs (3x0.74% = 2.22%).
Perceived gender | mIoU | stdev | Number of images |
---|---|---|---|
Female | 78.01% | 15.08% | 2585 |
Male | 79.05% | 13.96% | 3065 |
Average (across all images) | 78.55% | 14.55% | 5650 |
Average (across genders) | 78.53% | 0.74% | - |
Ethical Considerations
- Privacy: When the model is used in ARDK, inference is only applied on-device and the image is not transferred off the user device.
- Human Life: This model is designed for entertainment purposes within an augmented reality application. It is not intended to be used for making human life-critical decisions.
- Bias: Training datasets have not been audited for diversity and may present biases not surfaced by our benchmarks.
Caveats and Recommendations
- Our annotated dataset only contains binary genders, which we include as male/female. Further data would be needed to evaluate across a spectrum of genders.
- An ideal skin tone evaluation dataset would additionally include camera details, and more environment details such as lighting and humidity. Furthermore, the Fitzpatrick scale has limitations as it doesn't fully represent the full spectrum of human skin tones.
- This model card is based on the work of Mitchell, Margaret, et al. "Model cards for model reporting." Proceedings of the conference on fairness, accountability, and transparency. 2019. Link